Chains
- 四种通用链的使用
LLMChain
最常用的链
from langchain.chains import LLMChain
from langchain.llms import OpenAI
from langchain.prompts import PromptTemplate
llm = OpenAI(
temperature=0
)
prompt_template = "帮我给{product}想三个可以注册的域名?"
llm_chain = LLMChain(
llm=llm,
prompt=PromptTemplate.from_template(prompt_template),
verbose=True,#是否开启日志
)
#llm_chain({"product":"AI研习社"})
llm_chain("AI研习社")
顺序链 SimpleSequentialChain & SequentialChain
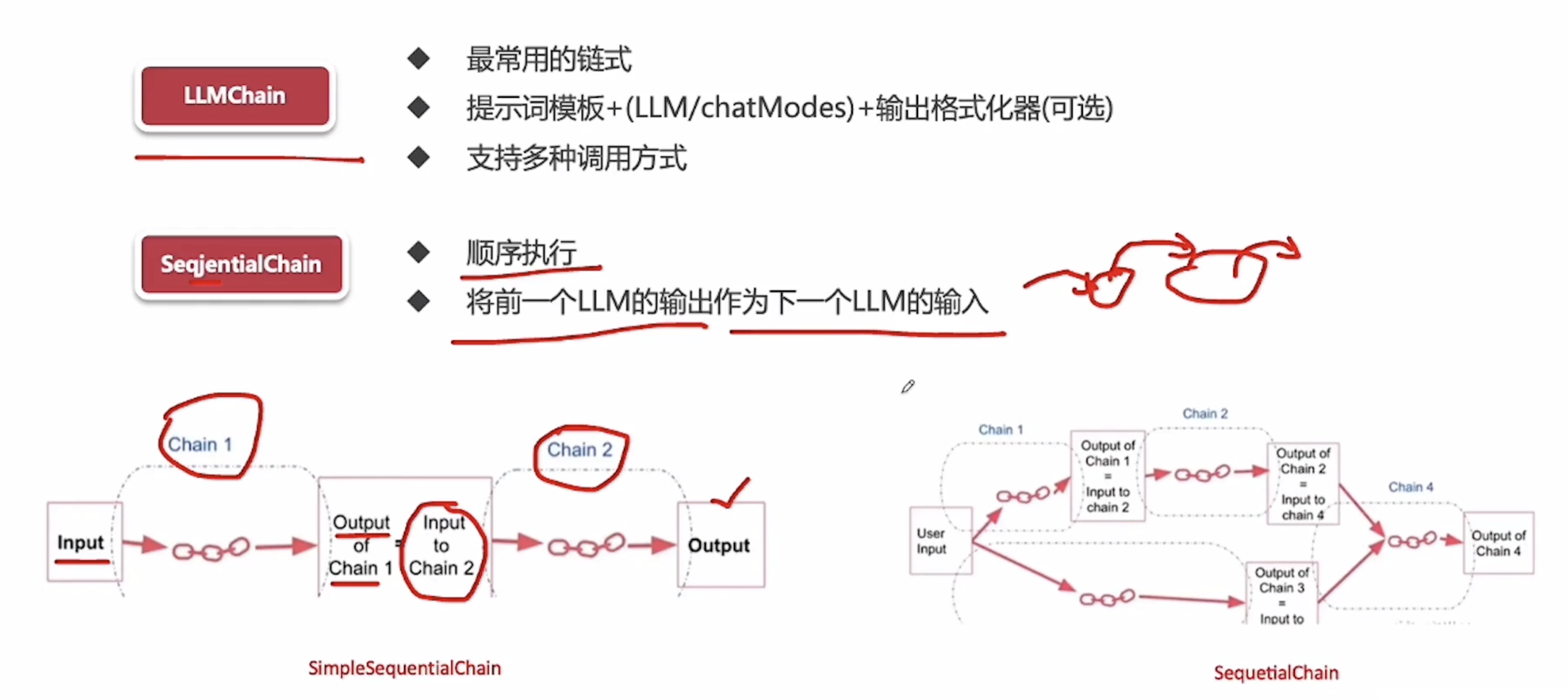
#simpleSequentialChain 只支持固定的链路
from langchain.chains import LLMChain
from langchain.chat_models import ChatOpenAI
from langchain.prompts import ChatPromptTemplate
from langchain.chains import SimpleSequentialChain
chat_model = ChatOpenAI(
temperature=0,
model="gpt-3.5-turbo",
)
#chain 1
first_prompt = ChatPromptTemplate.from_template("帮我给{product}的公司起一个响亮容易记忆的名字?")
chain_one = LLMChain(
llm=chat_model,
prompt=first_prompt,
verbose=True,
)
#chain 2
second_prompt = ChatPromptTemplate.from_template("用5个词来描述一下这个公司名字:{company_name}")
chain_two = LLMChain(
llm=chat_model,
prompt=second_prompt,
verbose=True,
)
overall_simple_chain = SimpleSequentialChain(
chains=[chain_one, chain_two],
verbose=True,#打开日志
)
overall_simple_chain.run("AI教育培训机构")
#SequentialChain 支持多个链路的顺序执行
# chain 1 -> chain 2 --> chain 4
# chain 3 --> chain 4
from langchain.chains import LLMChain
from langchain.chat_models import ChatOpenAI
from langchain.prompts import ChatPromptTemplate
from langchain.chains import SequentialChain
llm = ChatOpenAI(
temperature=0,
model="gpt-3.5-turbo",
)
#chain 1 任务:翻译成中文
first_prompt = ChatPromptTemplate.from_template("把下面内容翻译成中文:\n\n{content}")
chain_one = LLMChain(
llm=llm,
prompt=first_prompt,
verbose=True,
output_key="Chinese_Rview",
)
#chain 2 任务:对翻译后的中文进行总结摘要 input_key是上一个chain的output_key
second_prompt = ChatPromptTemplate.from_template("用一句话总结下面内容:\n\n{Chinese_Rview}")
chain_two = LLMChain(
llm=llm,
prompt=second_prompt,
verbose=True,
output_key="Chinese_Summary",
)
#chain 3 任务:智能识别语言 input_key是上一个chain的output_key
third_prompt = ChatPromptTemplate.from_template("下面内容是什么语言:\n\n{Chinese_Summary}")
chain_three = LLMChain(
llm=llm,
prompt=third_prompt,
verbose=True,
output_key="Language",
)
#chain 4 任务:针对摘要使用指定语言进行评论 input_key是上一个chain的output_key
fourth_prompt = ChatPromptTemplate.from_template("请使用指定的语言对以下内容进行回复:\n\n内容:{Chinese_Summary}\n\n语言:{Language}")
chain_four = LLMChain(
llm=llm,
prompt=fourth_prompt,
verbose=True,
output_key="Reply",
)
#overall 任务:翻译成中文->对翻译后的中文进行总结摘要->智能识别语言->针对摘要使用指定语言进行评论
overall_chain = SequentialChain(
chains=[chain_one, chain_two, chain_three, chain_four],
verbose=True,
input_variables=["content"],
output_variables=["Chinese_Rview", "Chinese_Summary", "Language", "Reply"],
)
RouterChain
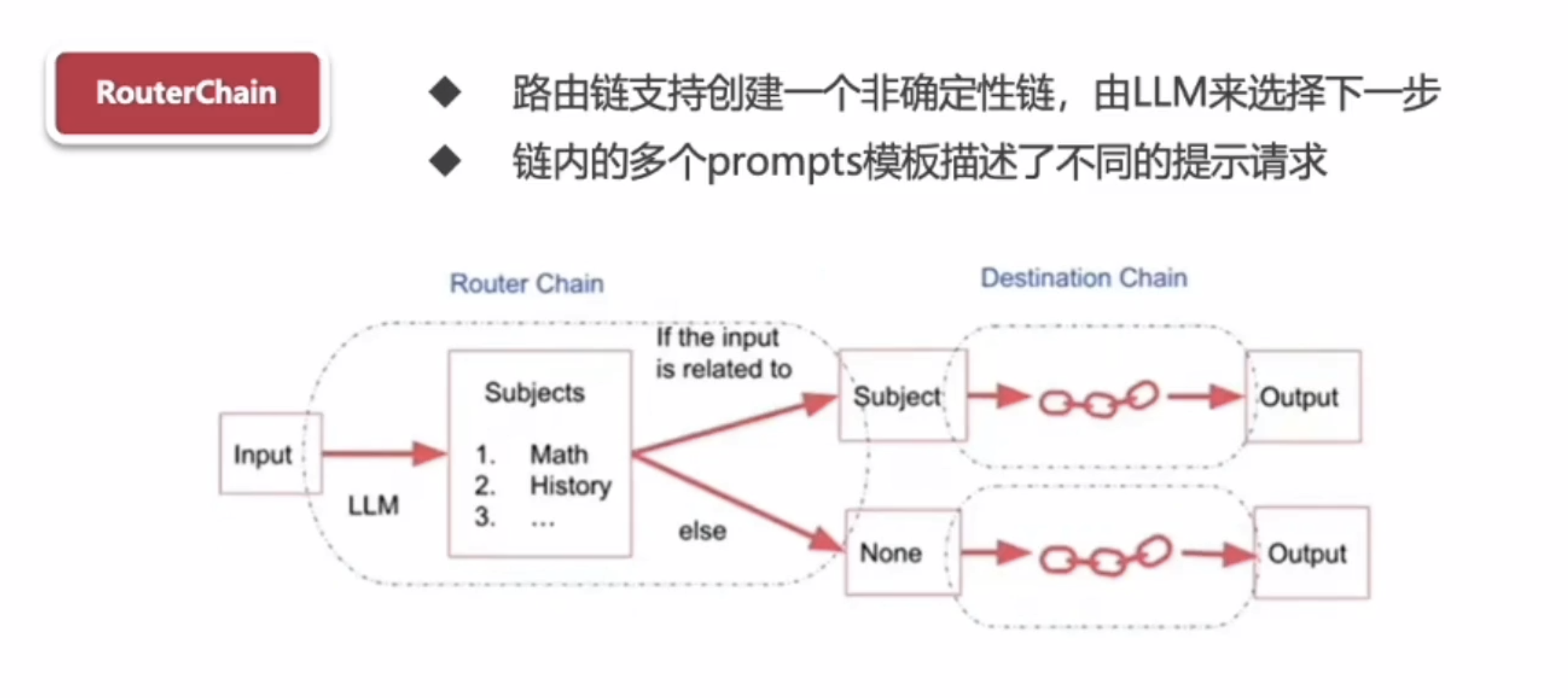
from langchain.prompts import PromptTemplate
#物理链
physics_template = """您是一位非常聪明的物理教授.\n
您擅长以简洁易懂的方式回答物理问题.\n
当您不知道问题答案的时候,您会坦率承认不知道.\n
下面是一个问题:
{input}"""
physics_prompt = PromptTemplate.from_template(physics_template)
#数学链
math_template = """您是一位非常优秀的数学教授.\n
您擅长回答数学问题.\n
您之所以如此优秀,是因为您能够将困难问题分解成组成的部分,回答这些部分,然后将它们组合起来,回答更广泛的问题.\n
下面是一个问题:
{input}"""
math_prompt = PromptTemplate.from_template(math_template)
from langchain.chains import ConversationChain
from langchain.chains import LLMChain
from langchain.llms import OpenAI
prompt_infos = [
{
"name":"physics",
"description":"擅长回答物理问题",
"prompt_template":physics_template,
},
{
"name":"math",
"description":"擅长回答数学问题",
"prompt_template":math_template,
},
]
llm = OpenAI(
temperature = 0
)
destination_chains = {}
for p_info in prompt_infos:
name = p_info["name"]
prompt_template = p_info["prompt_template"]
prompt = PromptTemplate(
template=prompt_template,
input_variables=["input"]
)
chain = LLMChain(
llm=llm,
prompt=prompt,
)
destination_chains[name] = chain
default_chain = ConversationChain(
llm = llm,
output_key="text"
)
from langchain.chains.router.llm_router import LLMRouterChain,RouterOutputParser
from langchain.chains.router.multi_prompt_prompt import MULTI_PROMPT_ROUTER_TEMPLATE
from langchain.chains.router import MultiPromptChain
destinations = [f"{p['name']}:{p['description']}" for p in prompt_infos]
destinations_str = "\n".join(destinations)
router_template = MULTI_PROMPT_ROUTER_TEMPLATE.format(destinations=destinations_str)
#print(MULTI_PROMPT_ROUTER_TEMPLATE)
router_prompt = PromptTemplate(
template=router_template,
input_variables=["input"],
output_parser=RouterOutputParser()
)
router_chain = LLMRouterChain.from_llm(
llm,
router_prompt
)
chain = MultiPromptChain(
router_chain=router_chain,
destination_chains=destination_chains,
default_chain=default_chain,
verbose=True
)
chain.run("什么是牛顿第一定律?")
chain.run("2+2等于几?")
chain.run("两个黄鹂鸣翠柳,下一句?")
Transformation 文档转换链
with open("letter.txt") as f:
letters = f.read()
from langchain.prompts import PromptTemplate
from langchain.chains import (
LLMChain,
SimpleSequentialChain,
TransformChain
)
from langchain.llms import OpenAI
def transform_func(inputs:dict) -> dict:
text = inputs["text"]
shortened_text = "\n\n".join(text.split("\n\n")[:3])
return {"output_text":shortened_text}
#文档转换链
transform_chain = TransformChain(
input_variables=["text"],
output_variables=["output_text"],
transform=transform_func
)
template = """对下面的文字进行总结:
{output_text}
总结:"""
prompt = PromptTemplate(
input_variables=["output_text"],
template=template
)
llm_chain = LLMChain(
llm = OpenAI(),
prompt=prompt
)
#使用顺序链连接起来
squential_chain = SimpleSequentialChain(
chains=[transform_chain,llm_chain],
verbose=True
)
#print(letters)
squential_chain.run(letters)
链的五种运行方式
from langchain import (
PromptTemplate,
OpenAI,
LLMChain
)
prompt_template = "给做{product}的公司起一个名字?"
llm = OpenAI(
temperature=0
)
llm_chain = LLMChain(
llm=llm,
prompt=PromptTemplate.from_template(prompt_template),
verbose=True
)
#llm_chain("儿童玩具")
#llm_chain.run("儿童玩具")
#llm_chain.apply([
# {"product":"儿童玩具"},
#])
#a = llm_chain.generate([
# {"product":"儿童玩具"},
#])
#print(a)
llm_chain.predict(product="儿童玩具")